Using AI to Enhance Data Engineering and ETL – The Intelligent Data Accelerator
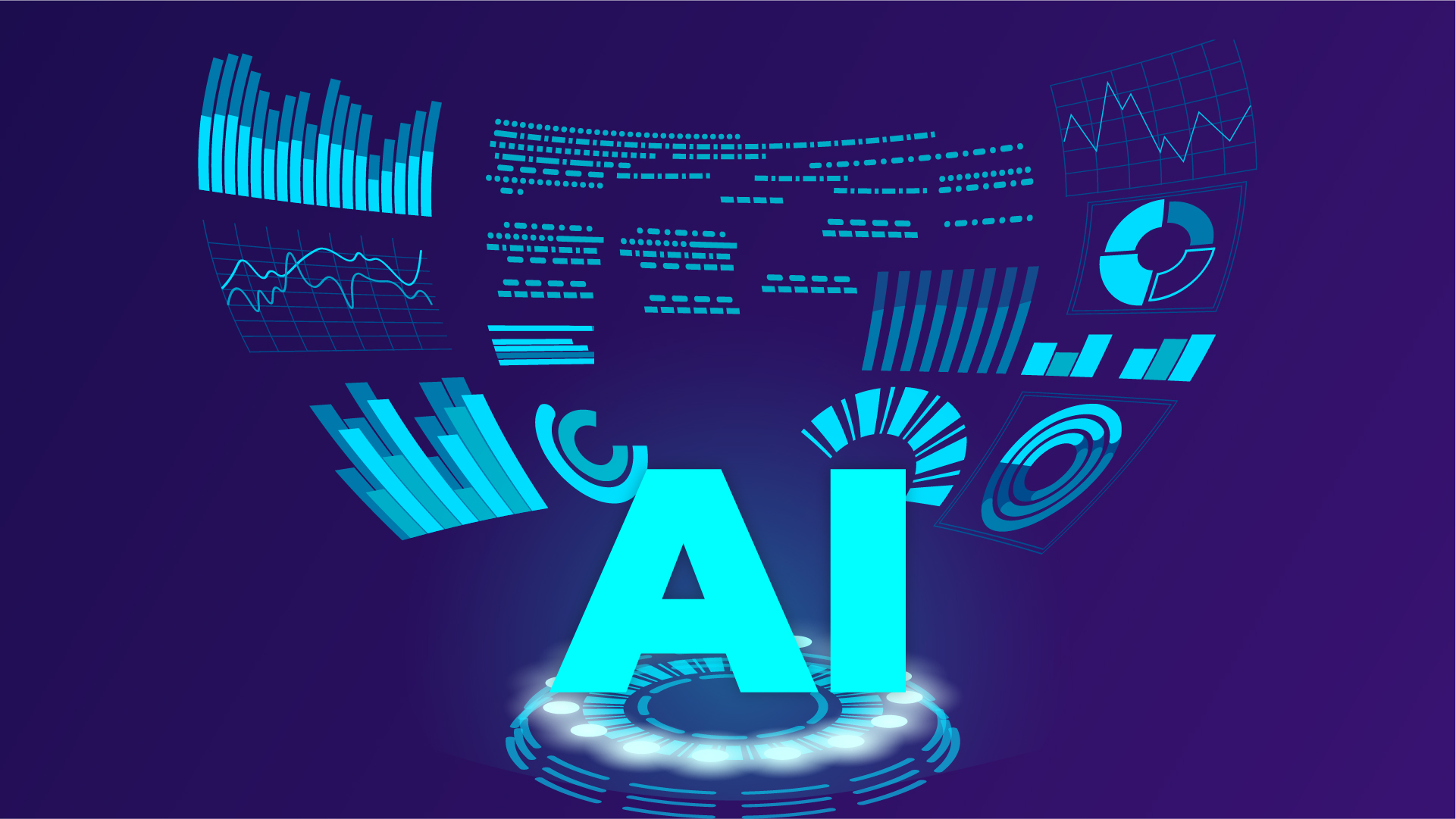
As data analytics becomes highly important to improve enterprise business performance, data aggregation (from across the enterprise and from outside sources) and adequate preparation of this data stand as critical phases within the analytics lifecycle.
An astonishing 40-60% of the overall effort in an enterprise is dedicated to these foundational processes.
It is here that the raw datasets are extracted from source systems, and cleaned, reconciled, and enriched before they can be used to generate meaningful insights for informed decision-making.
However, this phase often poses challenges due to its complexity and the variability of data sources.
Enter Artificial Intelligence (AI). It holds the potential to significantly enhance how we do data engineering and Extract, Transform, Load (ETL) processes. Check out our AI enabled ETL accelerator solution i.e. Intelligent Data Accelerator here.
In this blog, we delve into how AI can enhance data engineering and ETL management. We focus on its pivotal role in
- Setting up initial ETLs and
- Managing ongoing ETL processes efficiently.
AI-Powered Indirection to Bridge the Gap between Raw Data and ETL
AI introduces a remarkable concept of indirection between raw datasets and the actual ETL jobs, paving the way for increased efficiency and accuracy. We’ll address two major use cases hold promise to begin reshaping the data engineering landscape.
- Automating Initial ETL Setup through AI Training
Consider the scenario of media agencies handling large amounts of incoming client data about campaigns, click stream information, media information, and so on.
Traditionally, crafting ETL pipelines for such diverse data sources when new clients are onboarded can be time-consuming and prone to errors.
This is where AI comes to the rescue. By training AI models on historical ETL outputs, organizations can empower AI to scrutinize incoming datasets automatically.
The AI model adeptly examines the data, ensuring precise parsing and correct availability for ETL execution. For instance, an AI model trained on past campaigns’ performance data can swiftly adapt to new datasets, extracting crucial insights without manual intervention.
This leads to accelerated decision-making and resource optimization, exemplifying how AI-driven ETL setup can redefine efficiency for media agencies and beyond.
- AI Streamlining Ongoing ETL Management
The dynamic nature of certain datasets, such as insurance claims from diverse sources, necessitates constant adaptation of ETL pipelines.
Instead of manual intervention each time data sources evolve, AI can play a pivotal role. By employing AI models to parse and organize incoming data, ETL pipelines can remain intact while the AI handles data placement.
In the insurance domain, where claims data can arrive in various formats, AI-driven ETL management guarantees seamless ingestion and consolidation.
Even in our previous example where a media agency receives campaign data from clients, this data can frequently change as external systems change and new ones are added. AI can handle these changes easily, thus dramatically improving efficiency.
This intelligent automation ensures data engineers can focus on strategic tasks rather than reactive pipeline adjustments.
The result? Enhanced agility, reduced errors, and significant cost and time savings.
Domain-Specific Parsers: Tailoring AI for Precise Data Interpretation
To maximize the potential of AI in data engineering, crafting domain-specific parsers becomes crucial.
These tailored algorithms comprehend industry-specific data formats, ensuring accurate data interpretation and seamless integration into ETL pipelines.
From medical records to financial transactions, every domain demands a nuanced approach, and AI’s flexibility enables the creation of custom parsers that cater to these unique needs.
The combination of domain expertise and AI prowess translates to enhanced data quality, expedited ETL setup, and more reliable insights.
A Glimpse into the Future
As AI continues to evolve, the prospect of fully automating ETL management emerges.
Imagine an AI system that receives incoming data, comprehends its structure, and autonomously directs it to the appropriate target systems.
This vision isn’t far-fetched. With advancements in machine learning and natural language processing, the possibility of end-to-end automation looms on the horizon.
Organizations can potentially bid farewell to the manual oversight of ETL pipelines, ushering in an era of unparalleled efficiency and precision.
Next Steps
AI’s potential utility on data engineering and ETL processes is undeniable.
The introduction of AI-powered indirection revolutionizes how data is processed, from setting up initial ETLs to managing ongoing ETL pipelines.
The role of domain-specific parsers further enhances AI’s capabilities, ensuring accurate data interpretation across various industries.
Finally, as the boundaries of AI continue to expand, the prospect of complete ETL automation does not seem too far away.
Organizations that embrace AI’s transformative potential in this area stand to gain not only in terms of efficiency but also in their ability to accelerate insights generation.
Take a look at Ignitho’s AI enabled ETL accelerator which also includes domain specific partners. It can be trained in as little as a few weeks for your domain. Also read about Ignitho’s Intelligent Quality Accelerator, the AI powered IQA solution.